What is Negative Sampling in Retrieval?
A training technique where irrelevant data points are sampled to improve the performance of retrieval models.
More about Negative Sampling in Retrieval:
Negative Sampling in Retrieval is a method used to train retrieval models by introducing irrelevant examples (negatives) during the learning process. This helps the model differentiate between relevant and irrelevant data points, improving its ability to rank results accurately.
Negative sampling is a critical component in training tasks like dense retrieval, semantic search, and retrieval fusion, where distinguishing relevance is essential.
Frequently Asked Questions
Why is negative sampling important in retrieval training?
It helps models learn to identify irrelevant results, improving the precision of retrieval systems.
How are negative samples selected?
They can be selected randomly or through techniques like hard negative mining, which focuses on challenging examples.
From the blog
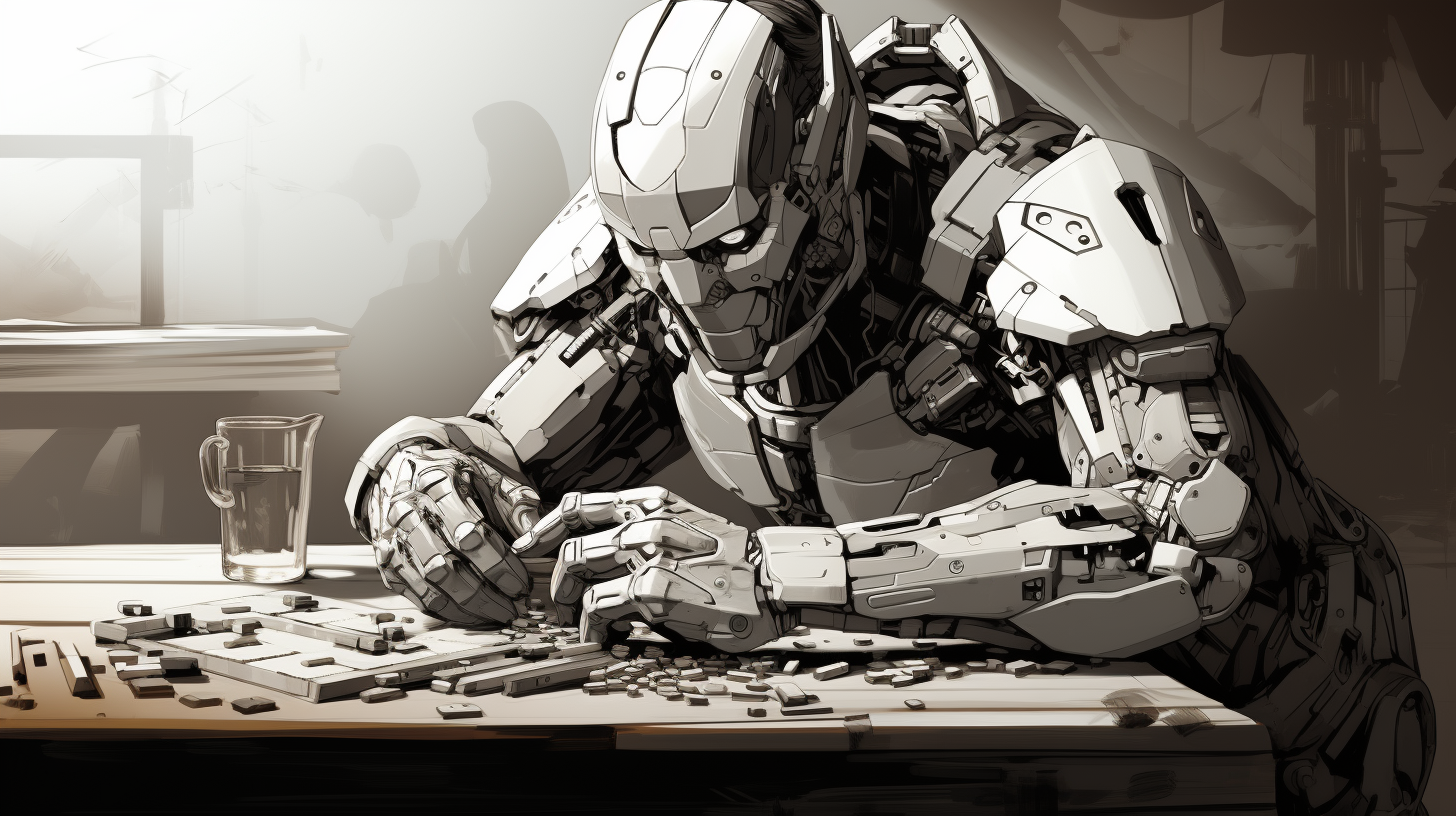
Enhancing ChatGPT with Plugins: A Comprehensive Guide to Power and Functionality
Explore the world of chatgpt plugins and how they empower chatbots with features like browsing, content creation, and more. Learn how SiteSpeakAI supports plugins to make its chatbots some of the most powerful available.
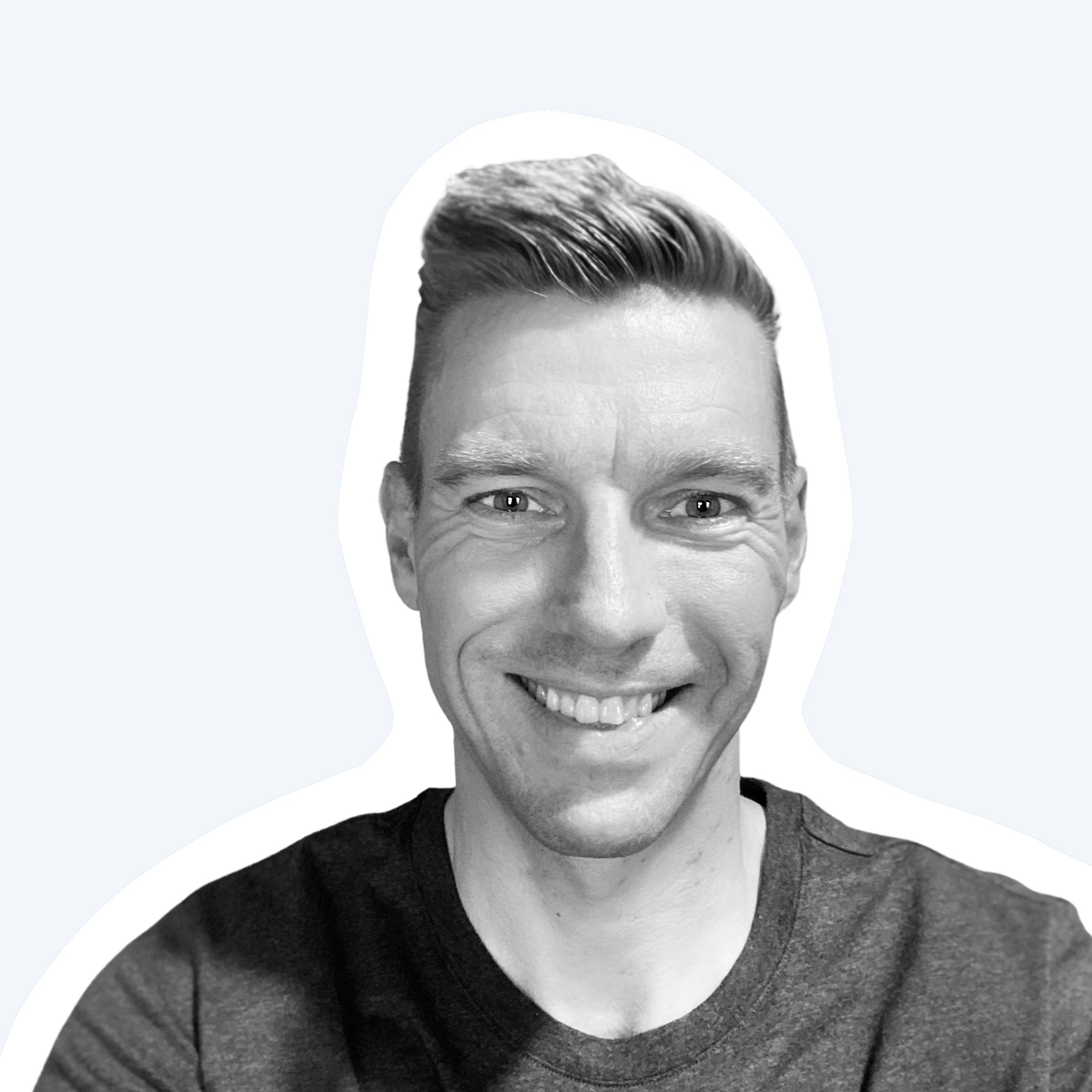
Herman Schutte
Founder
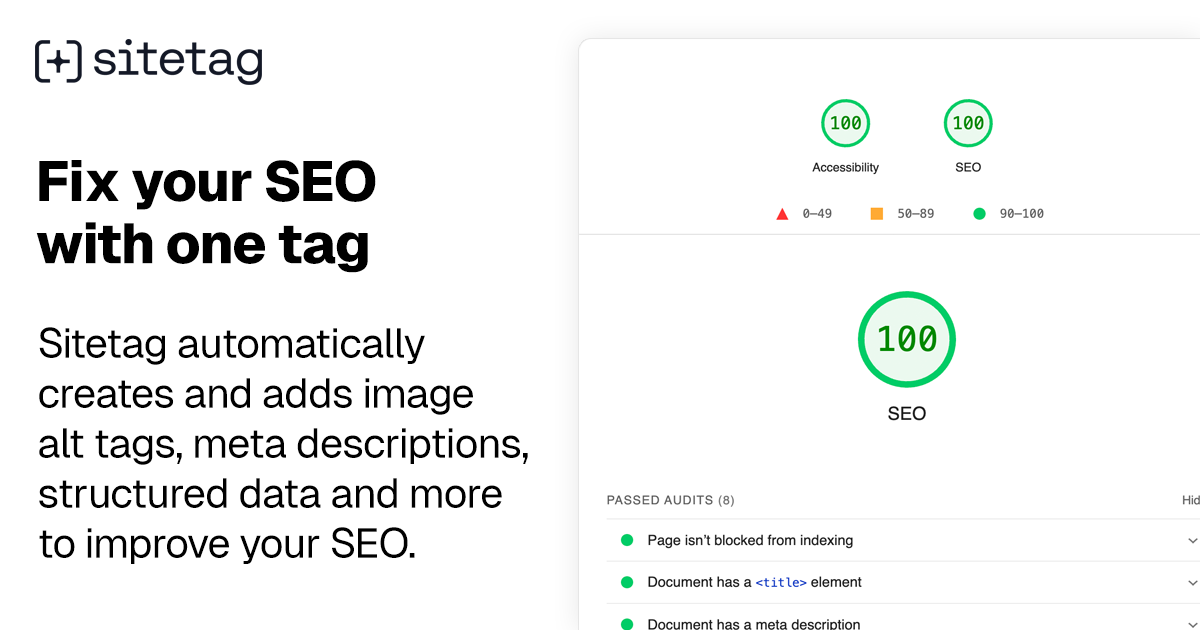
Fixing your Image Alt tags and SEO issues with AI
Optimizing your website's SEO can be complex and time-consuming, especially when it comes to image alt tags, title tags, and structured data. Sitetag, an AI-powered SEO tool, makes this process effortless. With just one script tag, Sitetag automatically enhances your website’s SEO elements, ensuring better search visibility and improved user experience—all without the manual work. Ready to simplify your SEO? Discover how Sitetag can transform your site today.
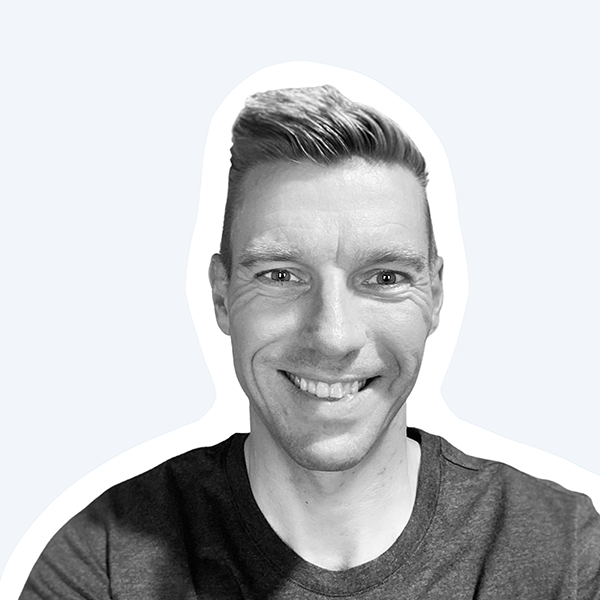
Herman Schutte
Founder