What is Retrieval Augmented Generation (RAG)?
A framework that combines retrieval and generation to produce responses grounded in external knowledge, leveraging techniques like dense retrieval and knowledge retrieval.
More about Retrieval Augmented Generation (RAG):
Retrieval Augmented Generation (RAG) is an AI framework that combines a retriever model with a generator model to produce responses grounded in external knowledge. The retriever fetches relevant documents or pieces of information using methods like dense retrieval or semantic search, while the generator uses that information to create contextually accurate and fact-based outputs.
RAG frameworks are particularly effective for applications such as open-domain question answering, knowledge-grounded dialogue systems, and other knowledge-intensive tasks, ensuring outputs are accurate and well-grounded.
Frequently Asked Questions
How does RAG improve response accuracy in AI models?
It retrieves relevant knowledge from external sources, such as vector databases, ensuring generated responses are fact-based and accurate.
What are common applications of RAG?
RAG is commonly used in question answering systems, customer support bots, and applications requiring real-time knowledge retrieval.
From the blog
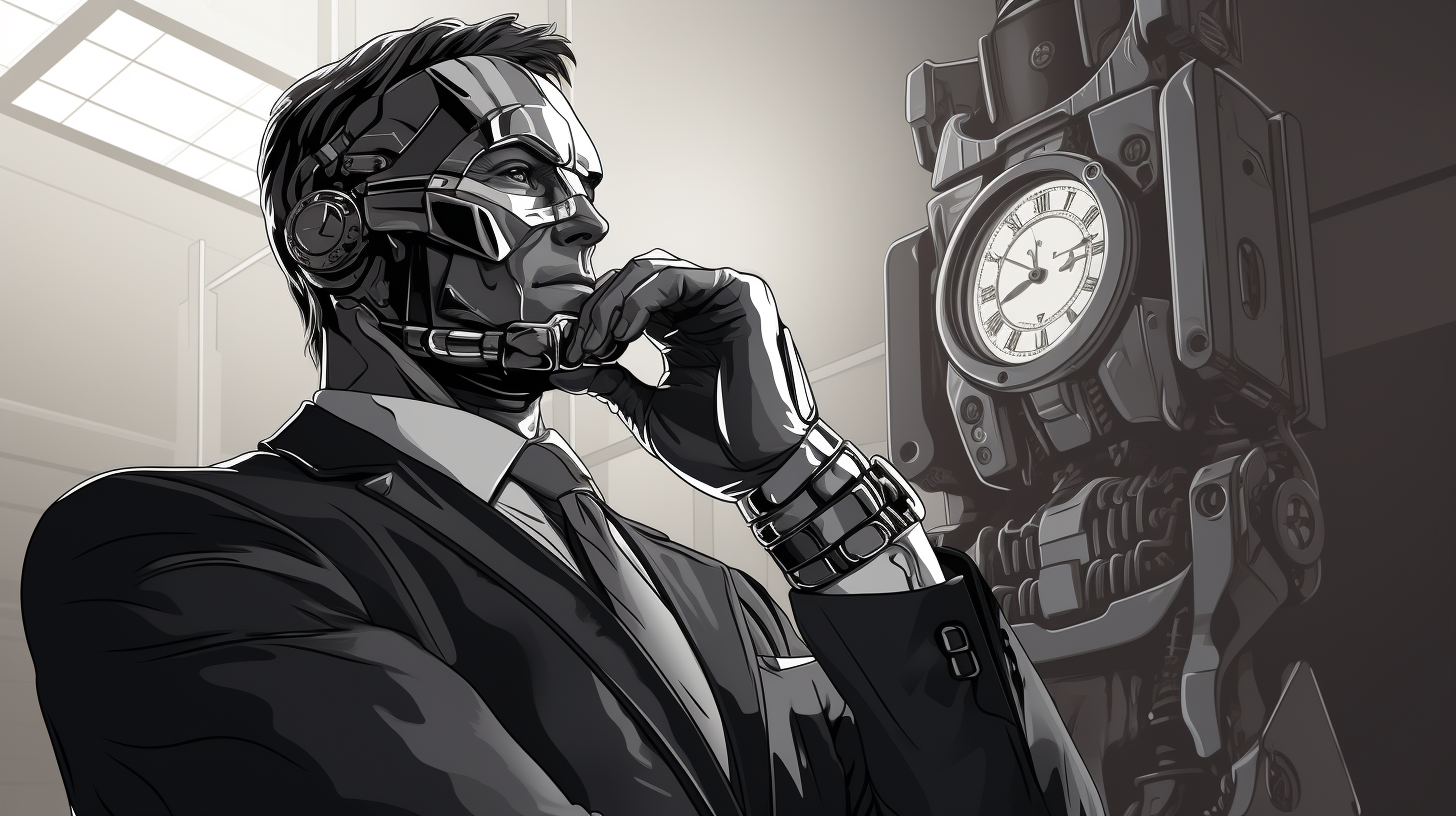
How AI Chatbots Can Save You 100s Of Hours In Customer Support
Dive into the transformative power of AI chatbots in customer support. Learn how businesses can save significant time and enhance customer satisfaction, with a look at tools like SiteSpeakAI.
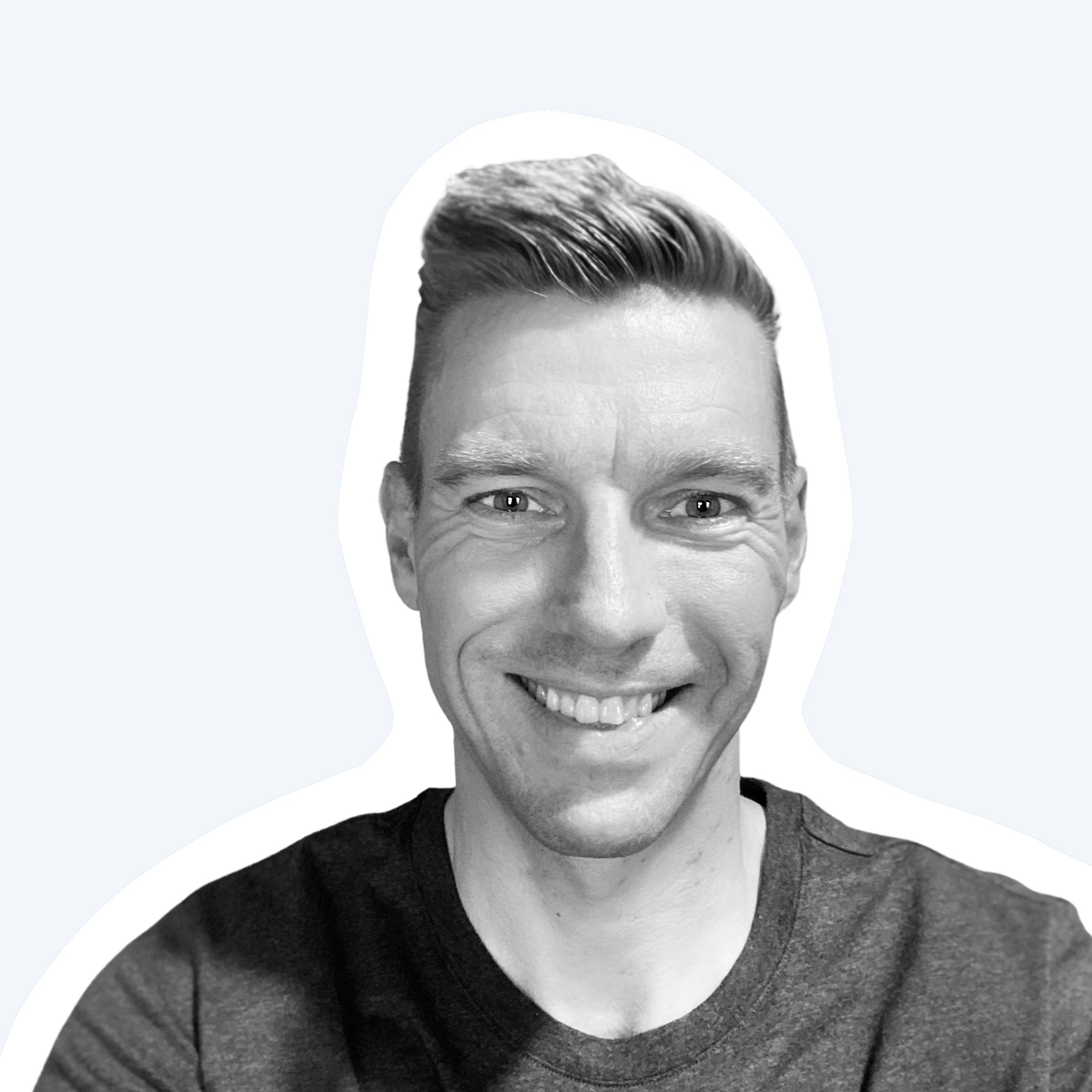
Herman Schutte
Founder
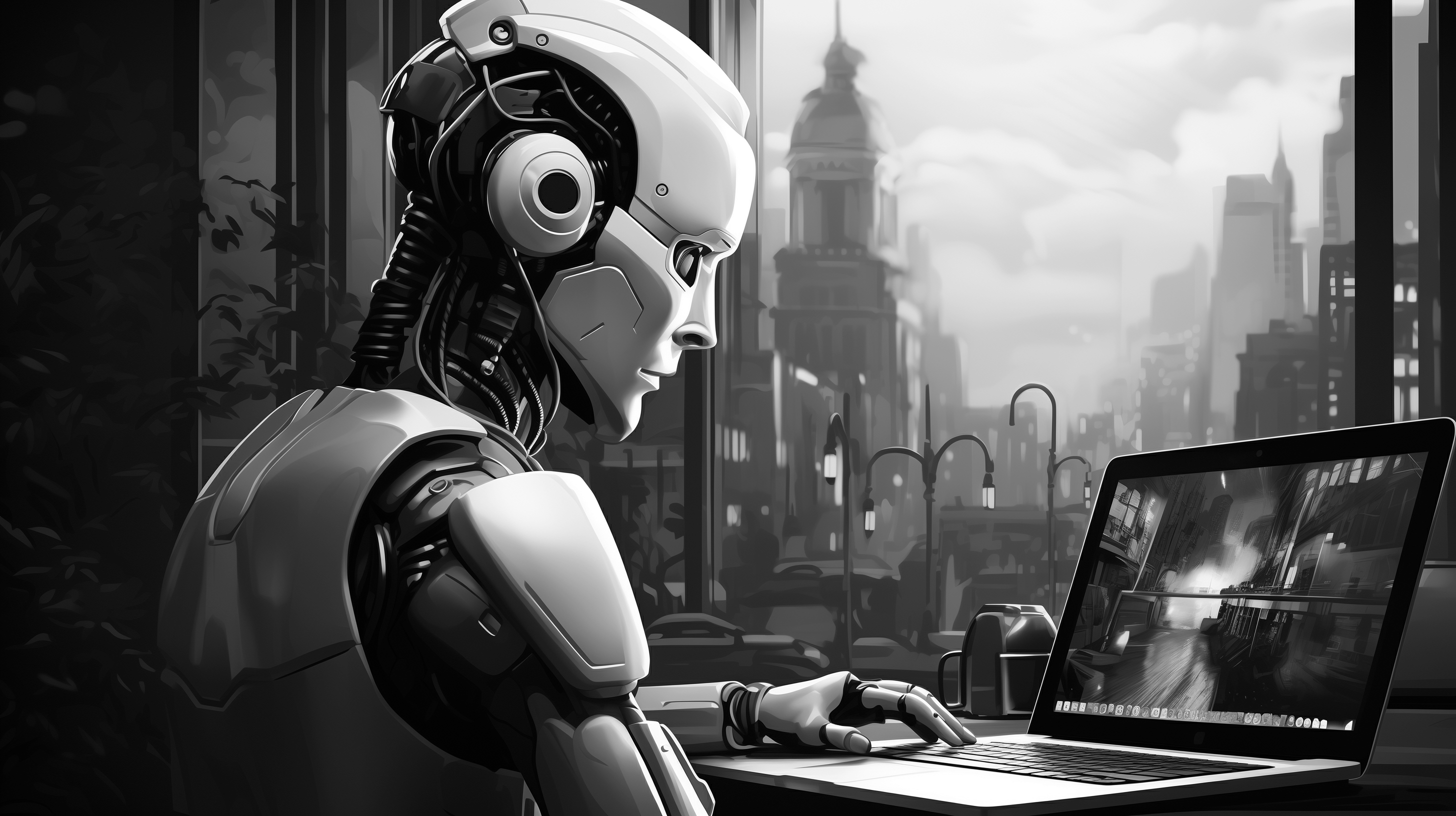
How SiteSpeakAI's YouTube Summarizer Can Transform Your Content Creation Strategy
Discover how SiteSpeakAI's YouTube Summarizer can revolutionize your content strategy. Learn to transform YouTube videos into SEO-optimized articles for your blog or website in under a minute. Boost engagement and search rankings effortlessly. Explore now.
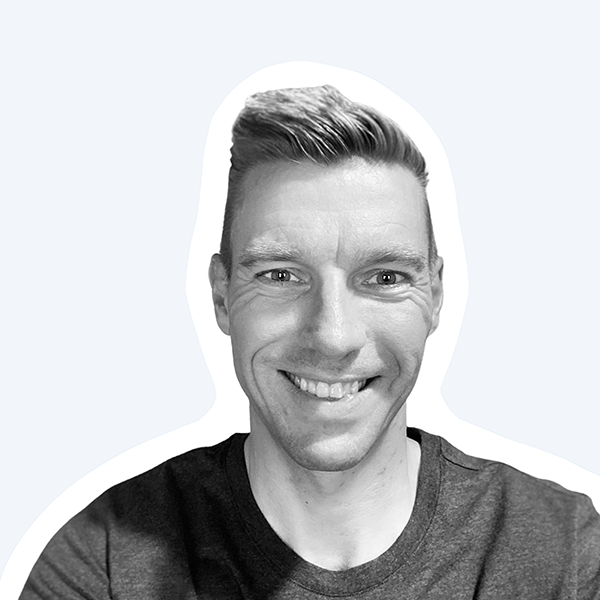
Herman Schutte
Founder