What is End-to-End Learning in AI?
A training approach where a system learns to map inputs directly to outputs, minimizing intermediate steps or feature engineering.
More about End-to-End Learning:
End-to-End Learning refers to training models where the entire process, from input to output, is learned directly from the data without manual intervention in feature extraction or intermediate representations. For instance, in a chatbot context, an end-to-end learning model might take user messages as input and produce chatbot responses as output without manually defined rules or intermediate processing.
This approach can be powerful as it allows the model to capture and learn intricate patterns in data. However, it often requires large amounts of data and can be more challenging to interpret or debug compared to models with more defined stages.
Frequently Asked Questions
What are the advantages of end-to-end learning?
End-to-end learning can lead to more robust and generalized models, especially when there is ample training data. It also reduces the need for manual feature engineering or domain-specific expertise.
Are there challenges associated with end-to-end learning?
End-to-end models can require significant data to train effectively. They can also be less transparent, making it harder to understand or debug their decisions.
From the blog
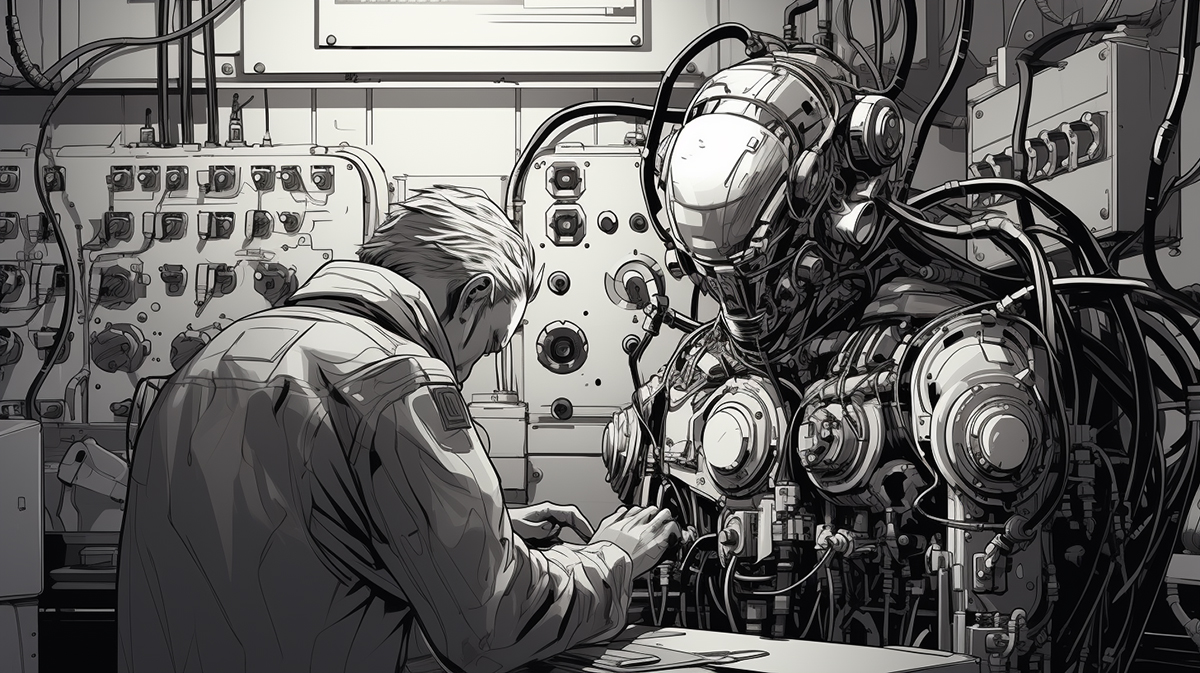
Fine-tuning your custom ChatGPT chatbot
Finetuning your custom chatbot is a crucial step in ensuring that it can answer your visitors questions correctly and with the best possible information.
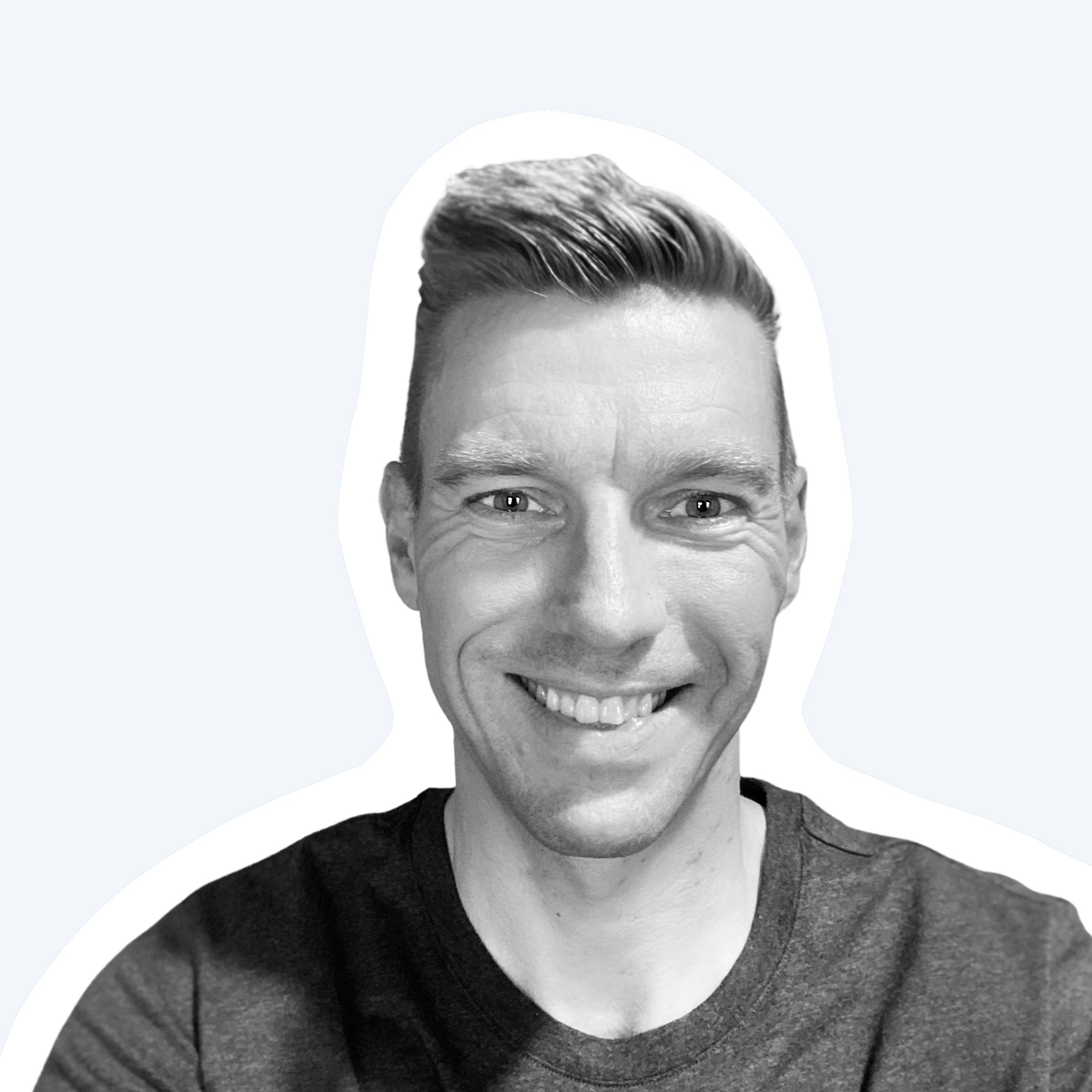
Herman Schutte
Founder
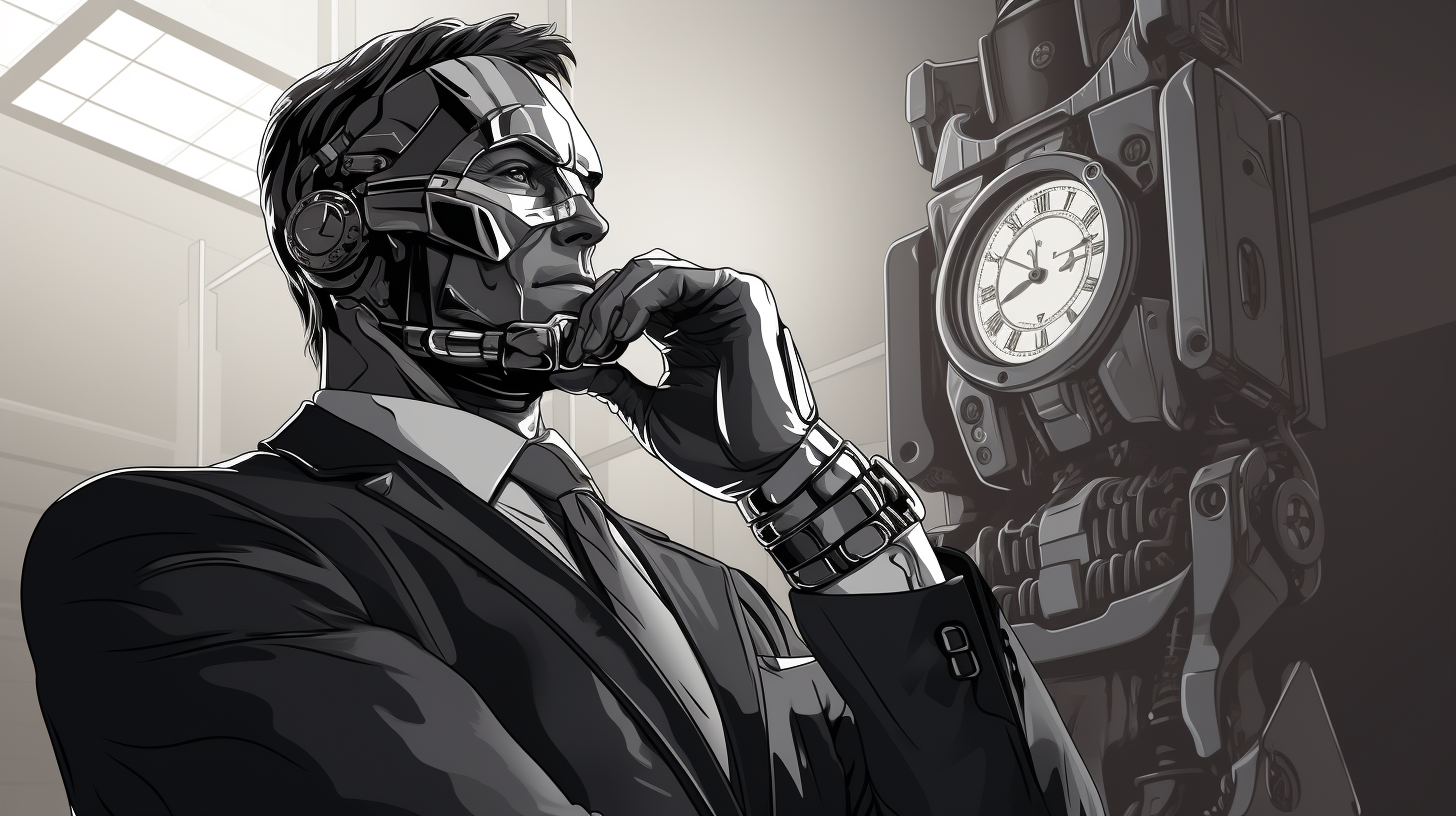
How AI Chatbots Can Save You 100s Of Hours In Customer Support
Dive into the transformative power of AI chatbots in customer support. Learn how businesses can save significant time and enhance customer satisfaction, with a look at tools like SiteSpeakAI.
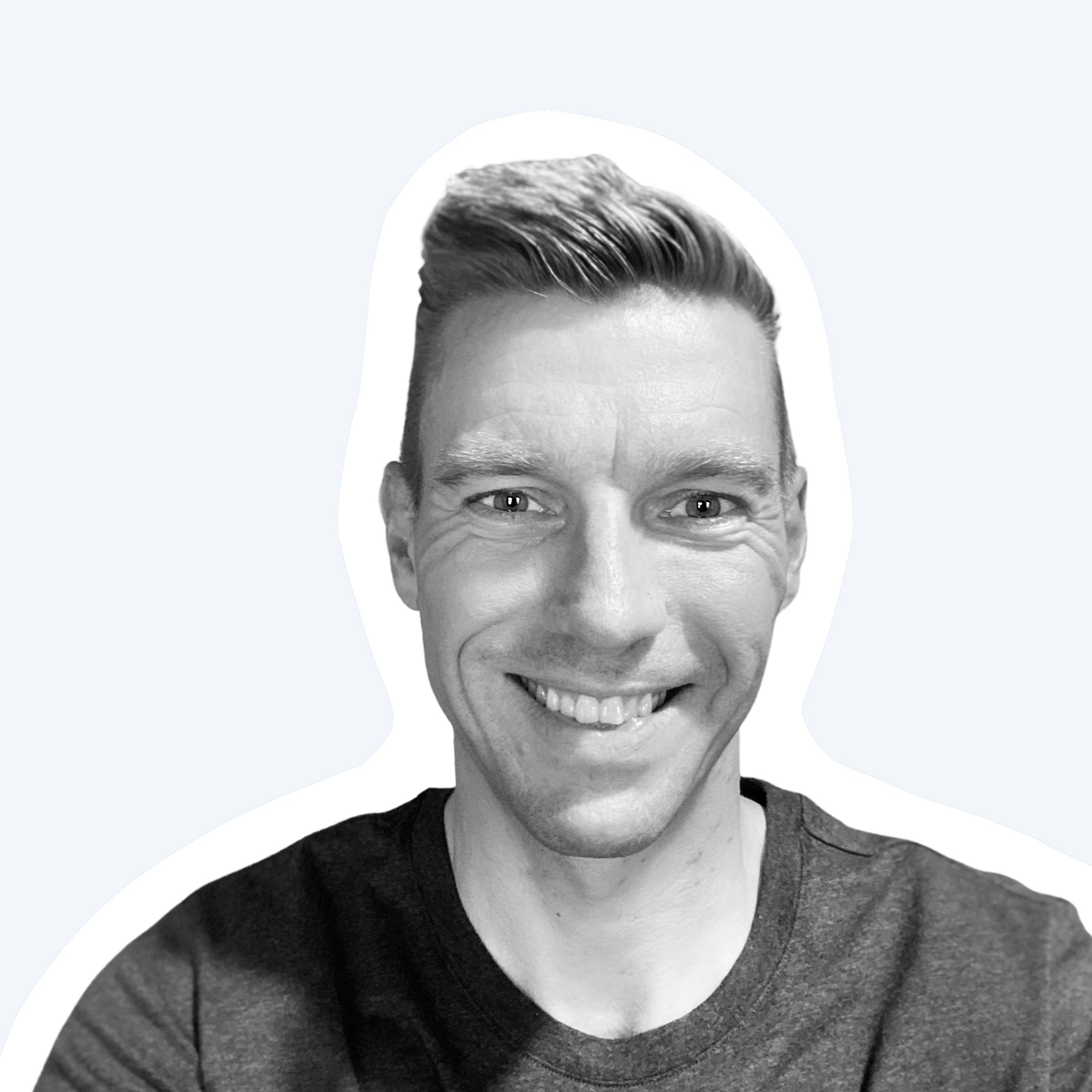
Herman Schutte
Founder