What is RAG Tokenization?
A tokenization method optimized for retrieval-augmented generation to balance efficiency and accuracy.
More about RAG Tokenization:
RAG Tokenization refers to the process of splitting input text into tokens specifically optimized for frameworks like retrieval-augmented generation (RAG). Proper tokenization ensures that retrieval and generation components interact efficiently, minimizing token limits while retaining contextual relevance.
This method is essential for balancing the context window size and accuracy in tasks like knowledge-grounded generation and context-aware generation.
Frequently Asked Questions
Why is RAG tokenization important?
It ensures optimal interaction between retrieval and generation components, improving the quality of outputs in RAG frameworks.
What challenges arise with RAG tokenization?
Challenges include managing token limits in the context window and ensuring retrieval efficiency.
From the blog
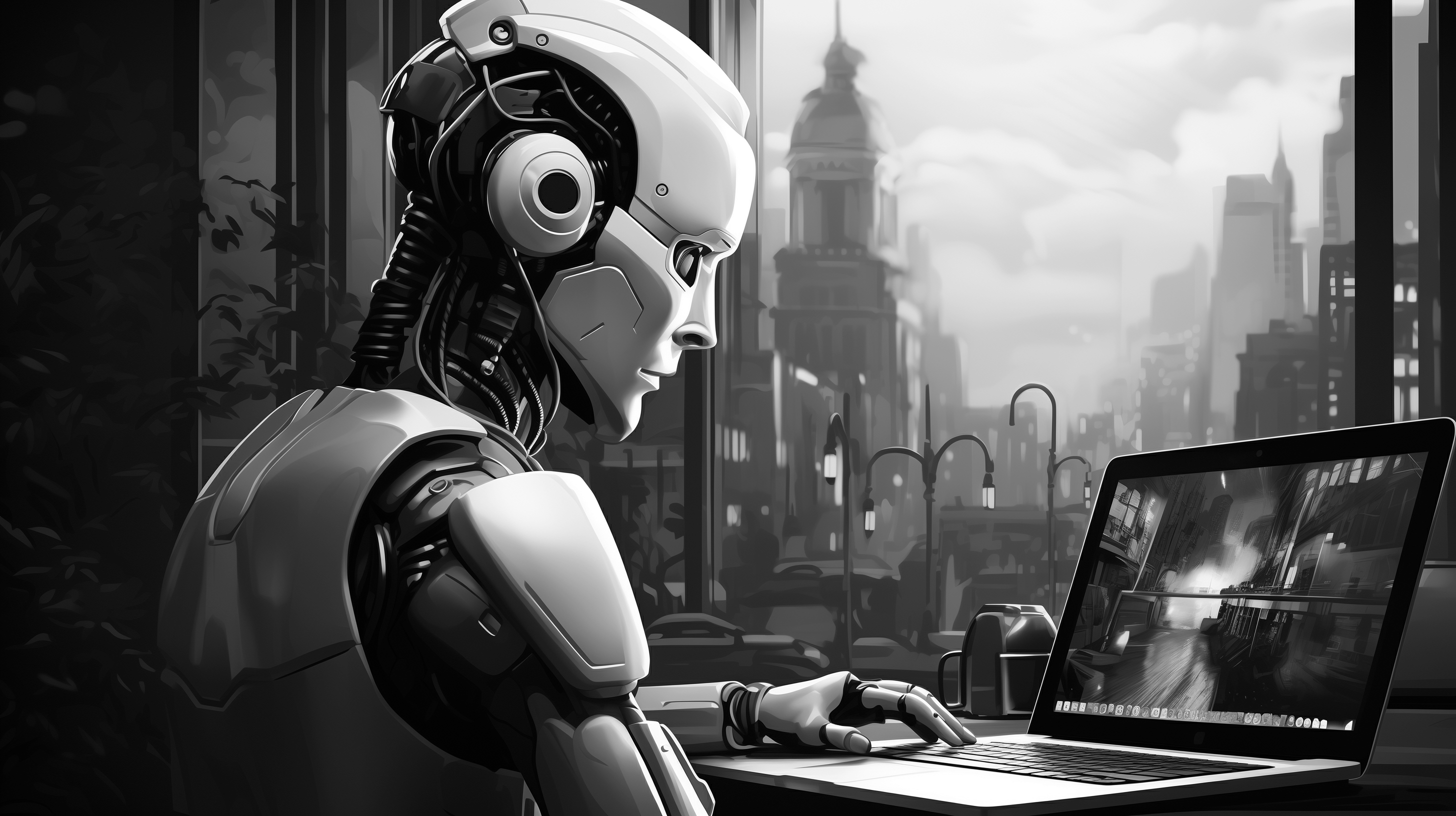
How SiteSpeakAI's YouTube Summarizer Can Transform Your Content Creation Strategy
Discover how SiteSpeakAI's YouTube Summarizer can revolutionize your content strategy. Learn to transform YouTube videos into SEO-optimized articles for your blog or website in under a minute. Boost engagement and search rankings effortlessly. Explore now.
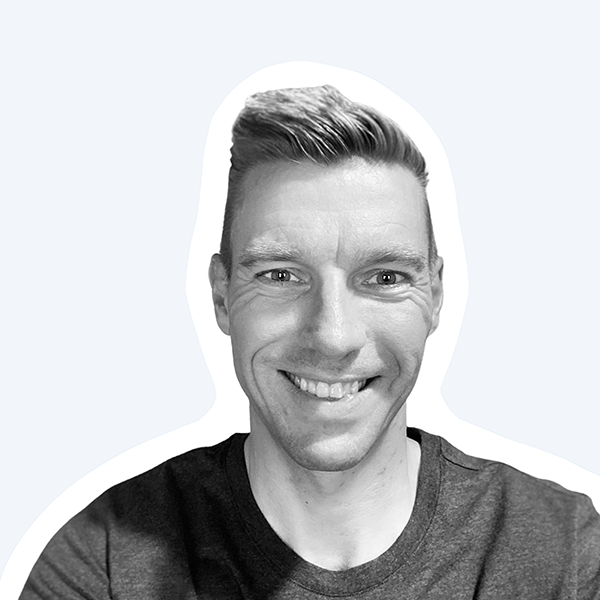
Herman Schutte
Founder
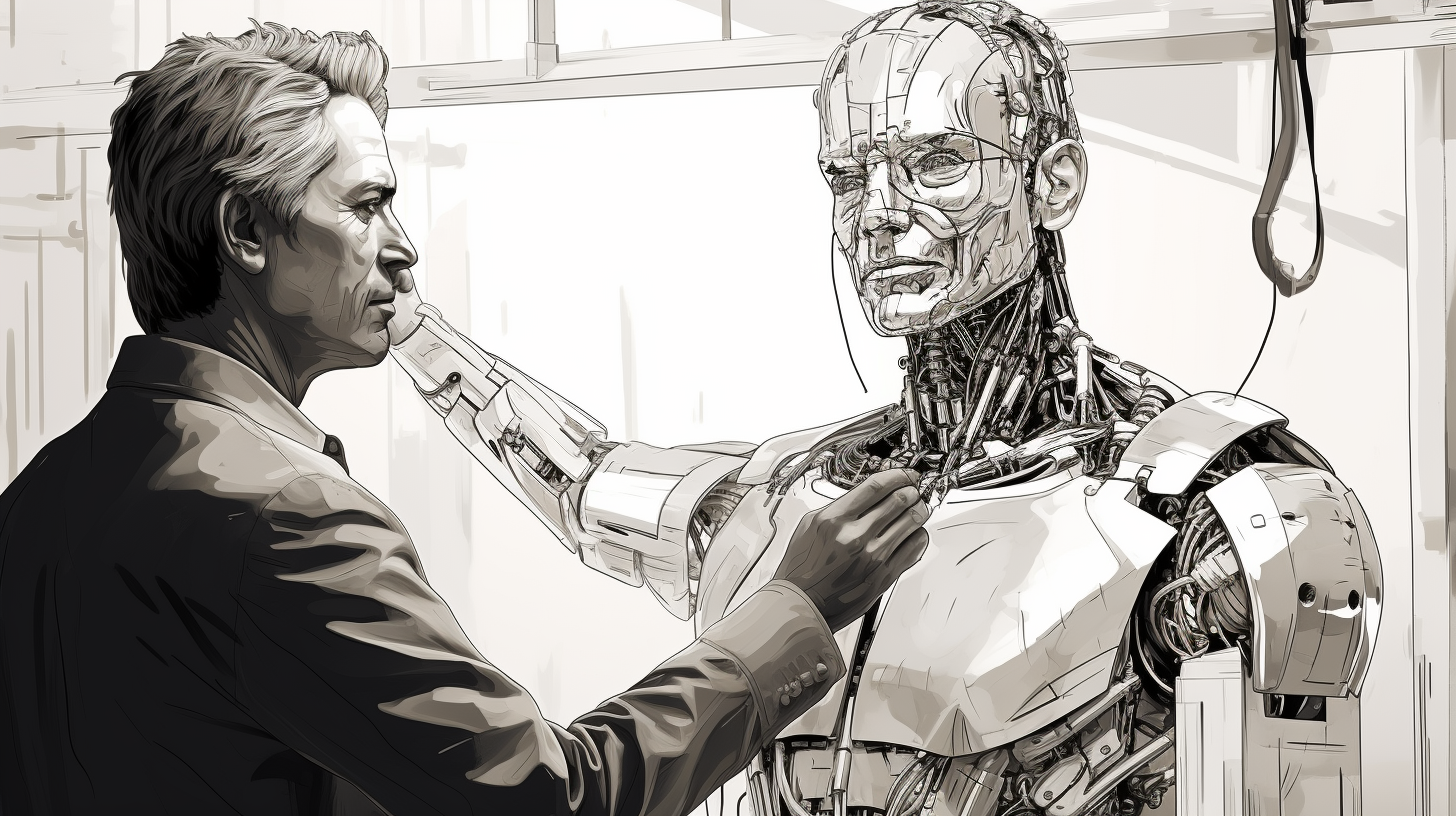
Custom model training and fine-tuning for GPT-3.5 Turbo
Today OpenAI announced that businesses and developers can now fine-tune GPT-3.5 Turbo using their own data. Find out how you can create a custom tuned model trained on your own data.
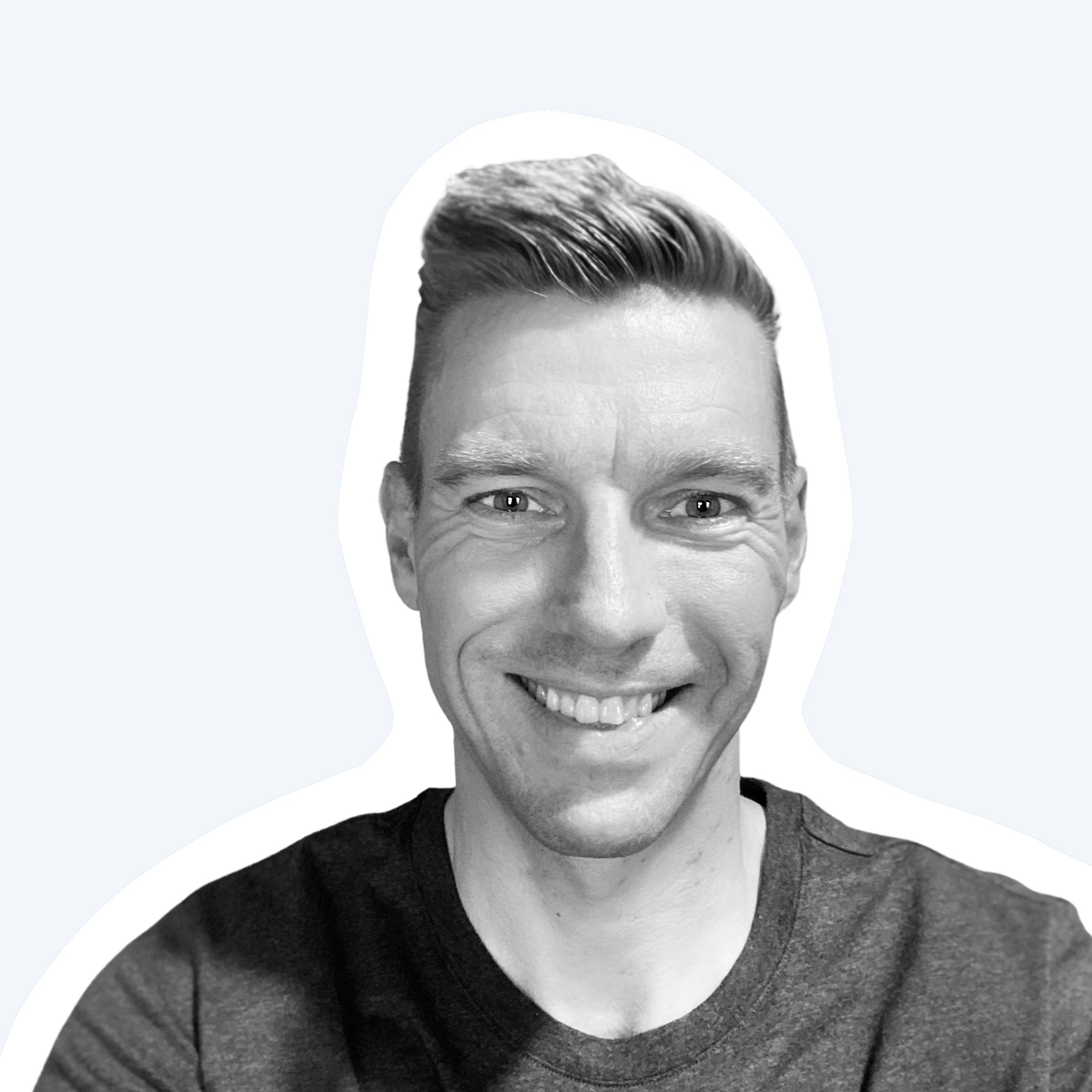
Herman Schutte
Founder