What are Token Embeddings?
Vector representations of individual tokens, such as words or subwords, used in language models.
More about Token Embeddings:
Token Embeddings are dense vector representations of individual tokens (e.g., words or subwords) in a high-dimensional space. These embeddings capture semantic relationships between tokens and are generated by models like BERT or GPT.
Token embeddings are foundational to tasks like semantic search, dense retrieval, and context-aware generation, where understanding token-level relationships is critical for performance.
Frequently Asked Questions
How are token embeddings generated?
They are generated by neural networks trained on large datasets, capturing semantic and syntactic token relationships.
What applications use token embeddings?
Applications include retrieval augmentation pipelines, knowledge retrieval, and document similarity.
From the blog
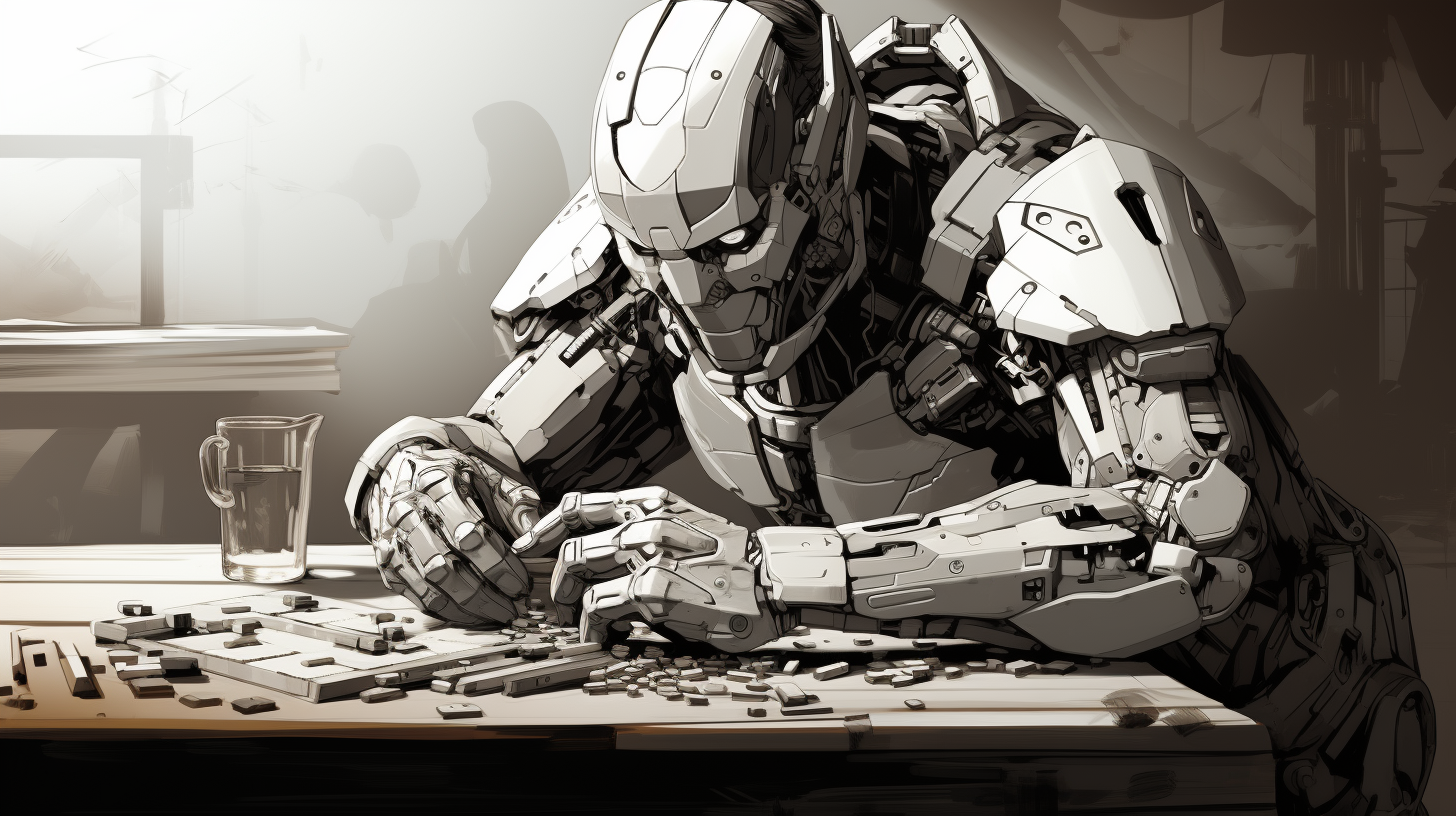
Enhancing ChatGPT with Plugins: A Comprehensive Guide to Power and Functionality
Explore the world of chatgpt plugins and how they empower chatbots with features like browsing, content creation, and more. Learn how SiteSpeakAI supports plugins to make its chatbots some of the most powerful available.
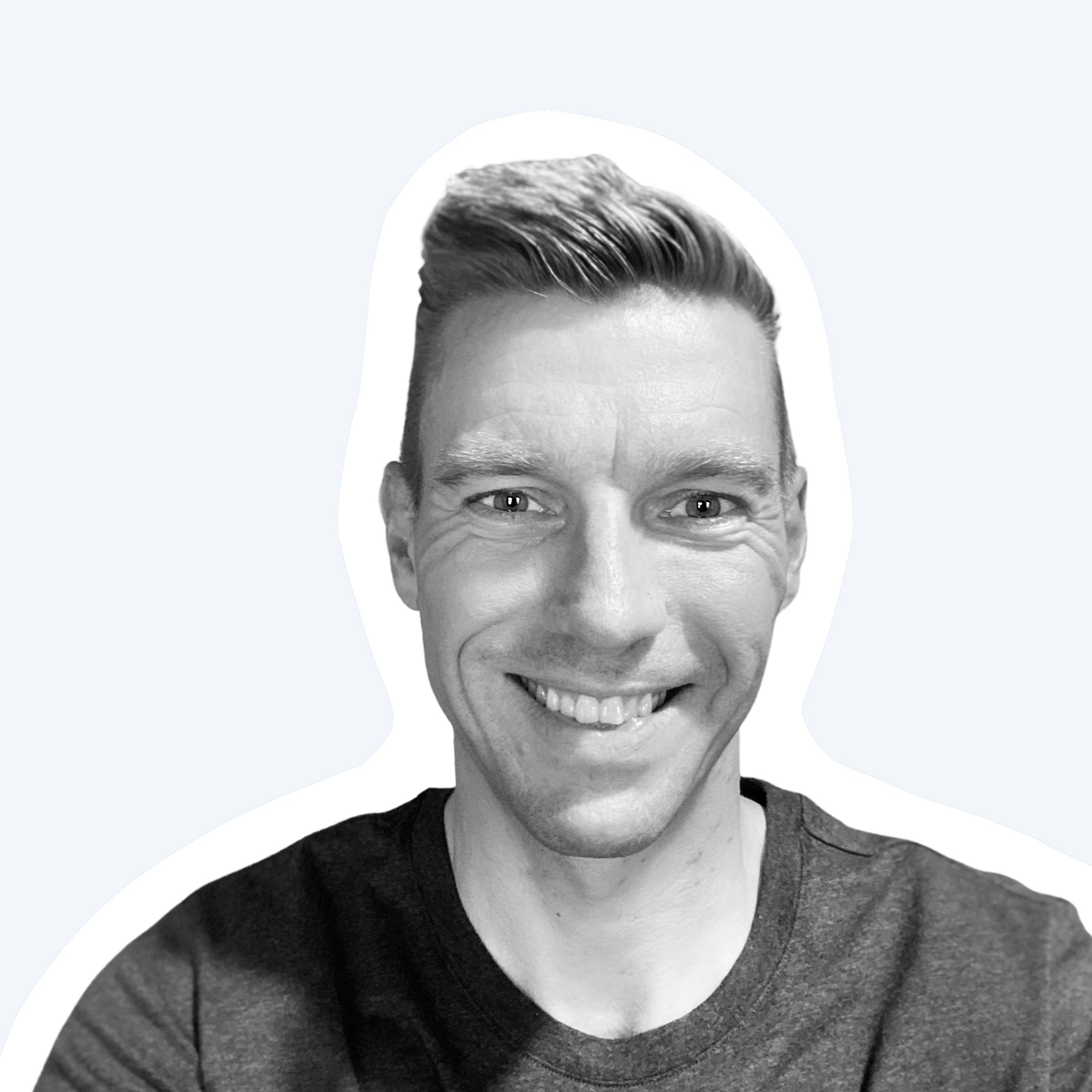
Herman Schutte
Founder
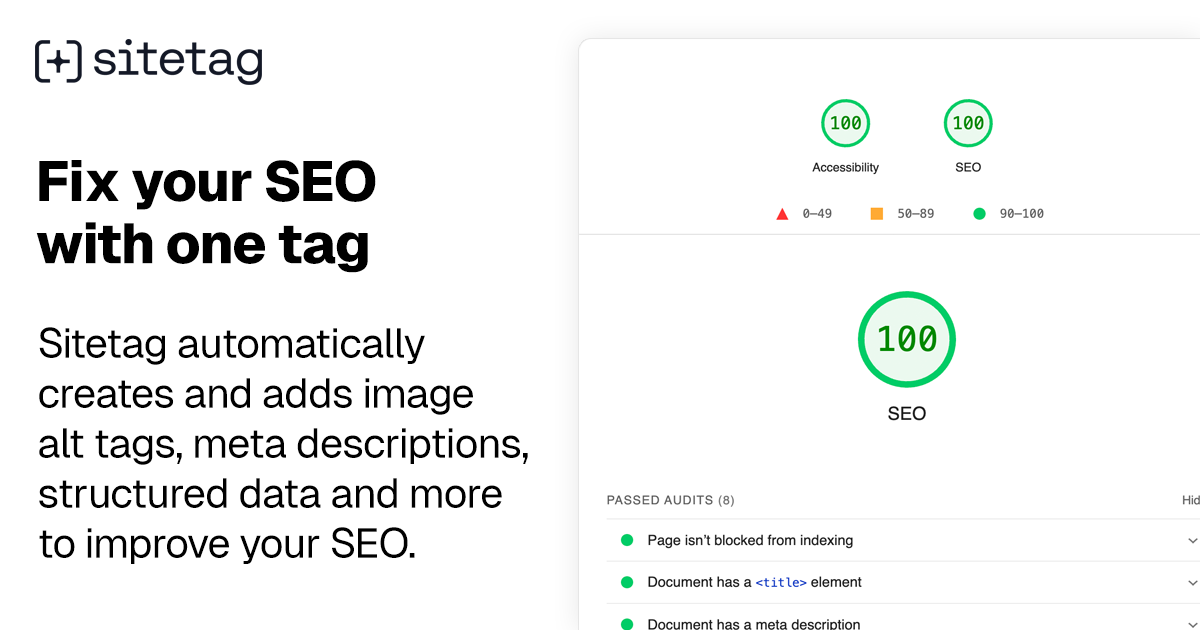
Fixing your Image Alt tags and SEO issues with AI
Optimizing your website's SEO can be complex and time-consuming, especially when it comes to image alt tags, title tags, and structured data. Sitetag, an AI-powered SEO tool, makes this process effortless. With just one script tag, Sitetag automatically enhances your website’s SEO elements, ensuring better search visibility and improved user experience—all without the manual work. Ready to simplify your SEO? Discover how Sitetag can transform your site today.
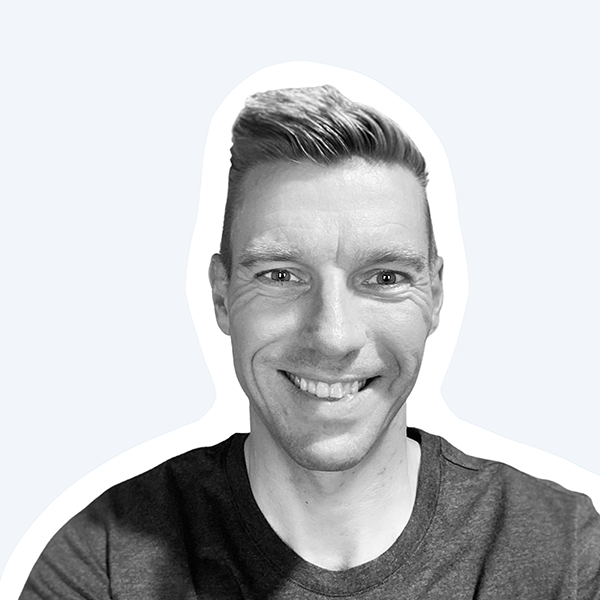
Herman Schutte
Founder